In the current era of AI and machine learning, the NVIDIA Tensor Core GPU stands out as a powerhouse, offering exceptional performance, intuitive operation, and top-quality security features to tackle AI and ML workloads. With its dedicated Transformer Engine specially designed to handle large language models and leveraging the cutting-edge NVIDIA Hopper architecture, it significantly boosts AI performance, delivering a remarkable 30x speed enhancement for large language models compared to previous versions. This advancement is truly exciting and promising for the future of AI technology!
Is this revolutionary NVIDIA H100 open to all?
Deciding whether to rent or buy an NVIDIA H100 GPU for your AI workload depends on several factors, including cost, usage duration, flexibility, and specific project needs. In this article, we will understand the nuances of each approach, which will help our readers make informed decisions that align with specific project requirements, budget constraints, and long-term goals.
Rent vs. Buy: Is Renting an NVIDIA H100 GPU the Right Choice for Your AI Workload?
In the rapidly evolving field of artificial intelligence, the demand for high-performance GPUs is higher than ever. The NVIDIA H100 GPU, renowned for its cutting-edge capabilities and unmatched performance, has become a key player in advancing AI research and development. However, with its significant cost, many AI professionals face a crucial decision: should they rent or buy this powerful hardware?
Let us see a few use-case scenarios!
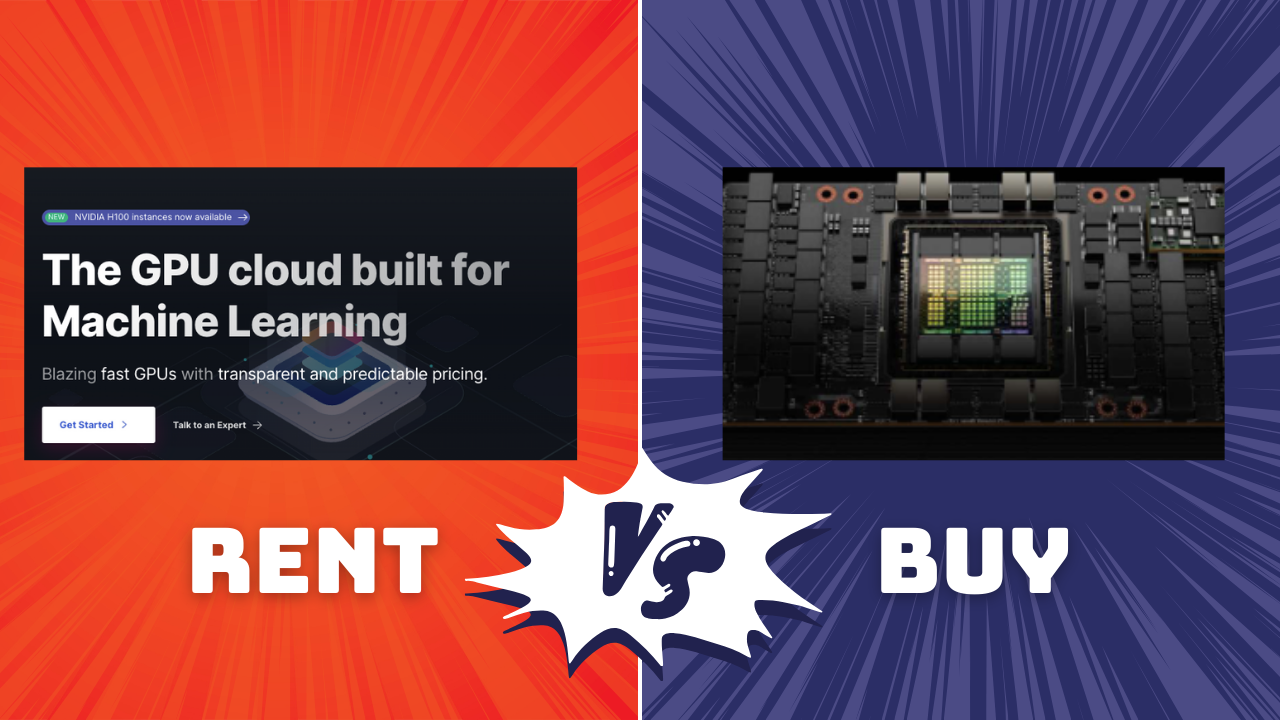
Scenario 1: Developing a Proof-of-Concept for a New AI Model
Imagine a company developing a proof-of-concept (PoC) for a new AI model that requires access to high-performance GPUs for a few months. Renting an NVIDIA H100 allows them to leverage top-tier hardware without the long-term financial commitment. Furthermore, purchasing top-tier hardware like the NVIDIA H100 would involve significant upfront costs and long-term commitments, which the company wants to avoid during this exploratory phase.
In this case, Paperspace's on-demand access to NVIDIA H100 GPUs will allow the company to rent the necessary hardware only for the duration of the PoC project. This eliminates the need for a large upfront investment and reduces financial risk.
As the PoC progresses, the company might need to scale up its computational resources. Paperspace’s cloud platform enables easy scaling, allowing the company to add more H100 GPUs as needed without any physical infrastructure changes.
Access to the latest NVIDIA H100 GPUs provides the company with cutting-edge performance for their AI workloads. This is particularly important for developing state-of-the-art AI models that require the best available hardware.
Paperspace’s user-friendly interface and seamless integration with popular AI frameworks and tools make it easy for the company’s developers to set up and manage their AI workloads. This reduces the setup time and allows the team to focus on developing and refining the AI model.
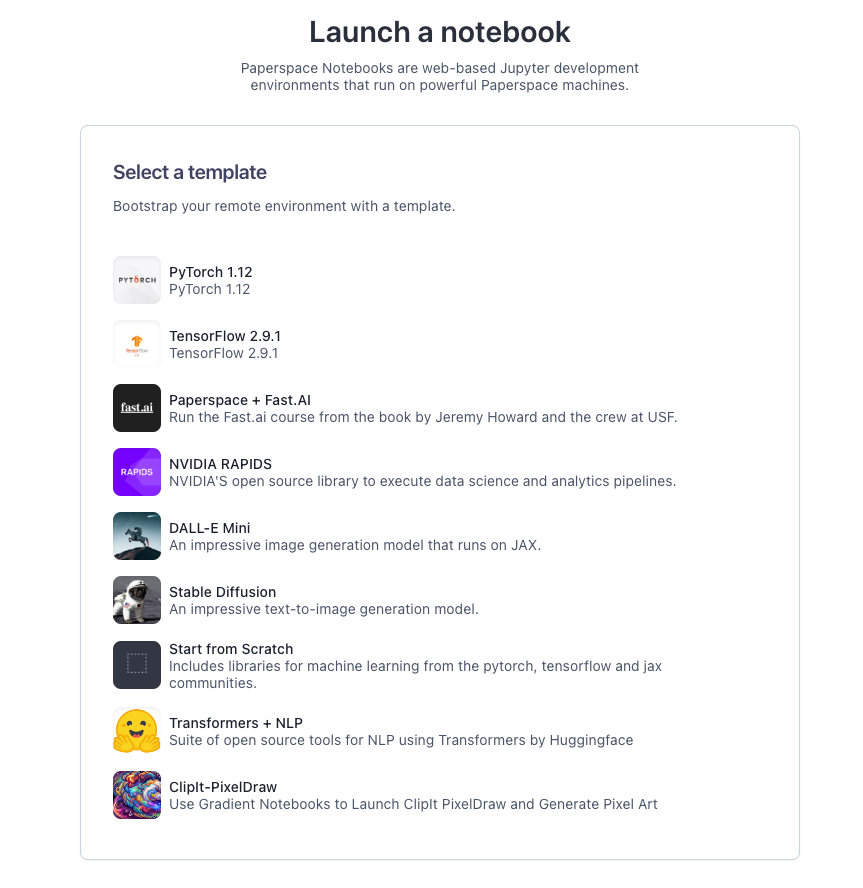
With Paperspace, the company benefits from ongoing maintenance and support for the rented hardware. This means they can rely on a stable, high-performing environment without worrying about hardware failures or technical issues.
Scenario 2: Research Lab with Fluctuating Computational Needs
A research lab is carrying out multiple projects that have varying computational requirements. During peak periods, when running complex simulations or processing large datasets, the lab requires extensive GPU resources. However, at other times, their computational needs are minimal. Investing in a large number of GPUs would lead to substantial idle hardware during low-demand periods, resulting in both cost and inefficiency.
Renting GPUs on Paperspace helps the lab avoid the high upfront costs associated with purchasing hardware. This pay-as-you-go model ensures that the lab’s budget is used efficiently, with expenses aligning directly with their computational needs.
The Paperspace platform offers an intuitive interface that makes it easy to manage GPU resources. Researchers can quickly allocate or deallocate GPUs, track usage, and adjust resources in real time to match their project requirements.
Paperspace enables collaborative work environments, allowing researchers to share resources and work together seamlessly, regardless of their location. This is particularly useful for large, multi-disciplinary projects that involve multiple teams.
Understanding the NVIDIA H100 GPU
The NVIDIA H100 GPU comes with impressive technical specifications, including advanced tensor cores and significantly enhanced performance over its predecessors. It's designed to handle the most demanding AI workloads, from deep learning to high-performance computing. With these capabilities, it's no wonder that the H100 is a go-to choice for AI practitioners aiming to push the boundaries of what's possible.
The H100 GPU comes with 14,592 CUDA cores and 80GB of HBM3 capacity with a 5,120-bit memory bus, which comes with a hefty price tag of around $30,000.
- The H100, built with 80 billion transistors, provides a significant advancement in AI acceleration, high-performance computing, memory bandwidth, interconnect, and data center communication.
- The NVIDIA H100 ensures high-performance security for workloads by providing hardware-based protection for data and applications through Confidential Computing.
The Transformer Engine, utilizing software and Hopper Tensor Core technology, accelerates training for transformer models, the key AI building block, by applying mixed FP8 and FP16 precisions to significantly speed up AI calculations.
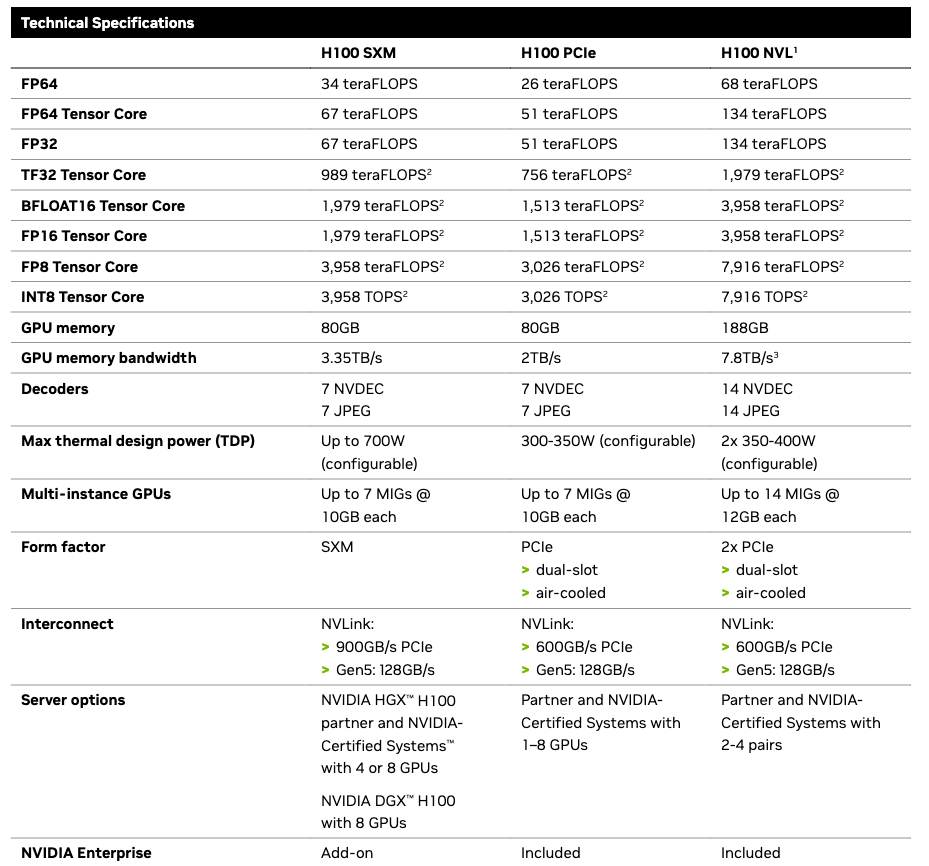
The NVIDIA GPUs, are equipped with fourth-generation Tensor Cores and FP8 precision in the Transformer Engine, allowing for up to 9X faster AI training and 30X faster inference for large language models. In terms of HPC, it triples FP64 FLOPS, introduces dynamic programming instructions for 7X higher performance, and supports secure multi-tenancy with second-generation MIG and built-in confidential computing.
Cost Analysis
Buying an NVIDIA H100 GPU involves substantial upfront costs. Beyond the purchase price, buyers must also account for the necessary infrastructure, such as cooling systems and power supply units. Additionally, ongoing costs include maintenance, upgrades, and operational expenses like electricity.
On the other hand, renting an NVIDIA H100 GPU can be more financially accessible. Paperspace offers flexible rental options, allowing users to scale resources according to their needs. This model eliminates the burden of upfront costs and infrastructure investment, making high-performance GPUs more accessible to a broader audience.
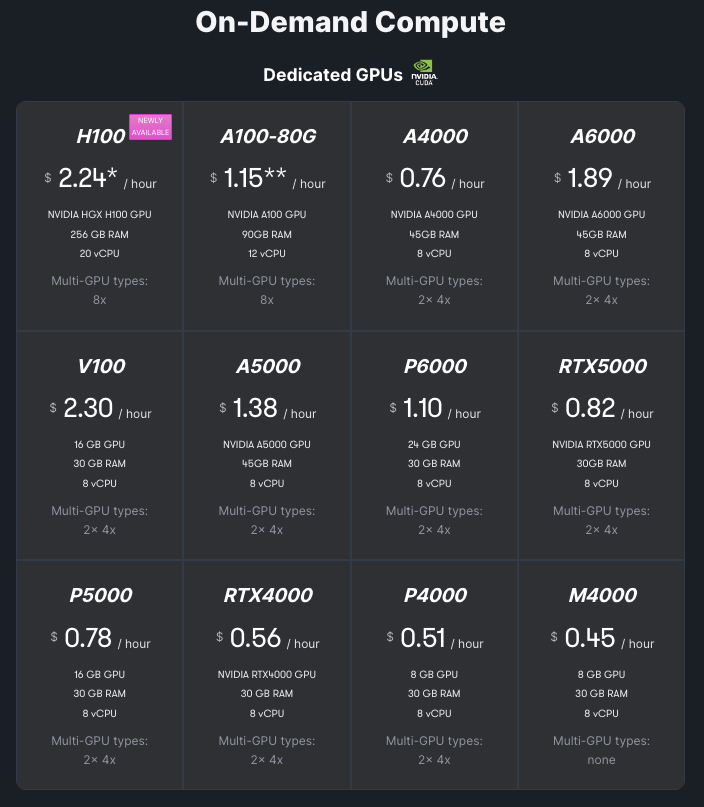
Pros and Cons of Buying
Pros | Cons |
---|---|
Once purchased, the hardware is yours to keep | Significant initial expenditure |
Complete control over the hardware and its usage | The hardware will depreciate over time |
Potential to revamp to newer GPU may come with some costs by selling the GPU later | Ongoing maintenance and operational management |
Pros and Cons of Renting
Pros | Cons |
---|---|
Reduced financial barriers to access high-performance hardware | Renting over a long period can become expensive |
Scale resources up or down based on project requirements | Relying on external services for hardware availability and performance |
Always have access to the most current hardware without the commitment | Potential restrictions on how the hardware can be used |
Paperspace customer team is always available to assist you with maintenance. Further, they are efficient in handling all maintenance, updates, and technical support, freeing the users to focus on other critical tasks |
Dependency on a third-party service provider for GPU availability, performance consistency, and support can impact workflow reliability |
Decision-Making Framework
When deciding between renting and buying, consider the following factors:
- Budget constraints: Evaluate financial resources and funding availability.
- Project duration: Determine whether the need for high-performance computing is short-term or long-term.
- Workload variability: Assess if computational demands will fluctuate significantly over time.
- Technical expertise: Ensure the necessary skills for hardware management and maintenance are available.
Conclusion
Choosing between renting and buying an NVIDIA H100 GPU depends on individual needs and circumstances. While renting offers flexibility and lower upfront costs, buying provides long-term value and control. AI professionals can make an informed decision by carefully assessing budget, project duration, and workload variability, that best suits their unique requirements.
By leveraging Paperspace, any startup or enterprise can efficiently manage its fluctuating computational needs, ensuring high performance during peak periods without incurring unnecessary costs during low-demand times. This flexibility and cost efficiency are crucial for advancing their research projects while maintaining budgetary control.