Josh Nicholson is the Co-Founder and CEO of scite (www.scite.ai), which is using deep learning to analyze the entirety of scientific literature to better measure the veracity of scientific work.
We were excited to sit down with him to learn more about his remarkable and ambitious machine learning project.
Bring this project to life
Paperspace: How did you come to create scite?
Nicholson: The idea for scite came from the observation that more often than not, cancer research could not be reproduced when tested independently. This problem exists in other fields as well, not just cancer research. We wanted to find a way to make scientific research more reliable and machine learning has enabled us to analyze the literature on a massive scale.
Paperspace: How many scientific articles have you performed analysis on so far?
Nicholson: We've analyzed 526,695,986 citation statements from 16,158,032 articles so far, and that number is climbing rapidly every day.
Paperspace: How can scite help readers evaluate a scientific article?
Nicholson: With scite, we’re working to introduce Smart Citations. These citations provide the context for each citation and its meaning. For example, we want to know whether the citation provides supporting or contradicting evidence – not just the metrics of how many times it has been previously cited, viewed, or downloaded. This allows people to look at a study and quickly determine if it has been supported or contradicted.
Paperspace: Where do you get the data to train your model and create the citation graph?
Nicholson: scite utilizes open access articles as well as content that is not open by partnering with leading academic publishers, like Wiley, IOP, Rockefeller University Press, Karger, BMJ, and others.
Paperspace: How does the model work?
Nicholson: After we identify the citation and its context, our deep learning model classifies the citation statement into one of three categories: supporting, contradicting or mentioning. This model has been trained on tens of thousands of manually annotated snippets from a diversity of scientific fields.
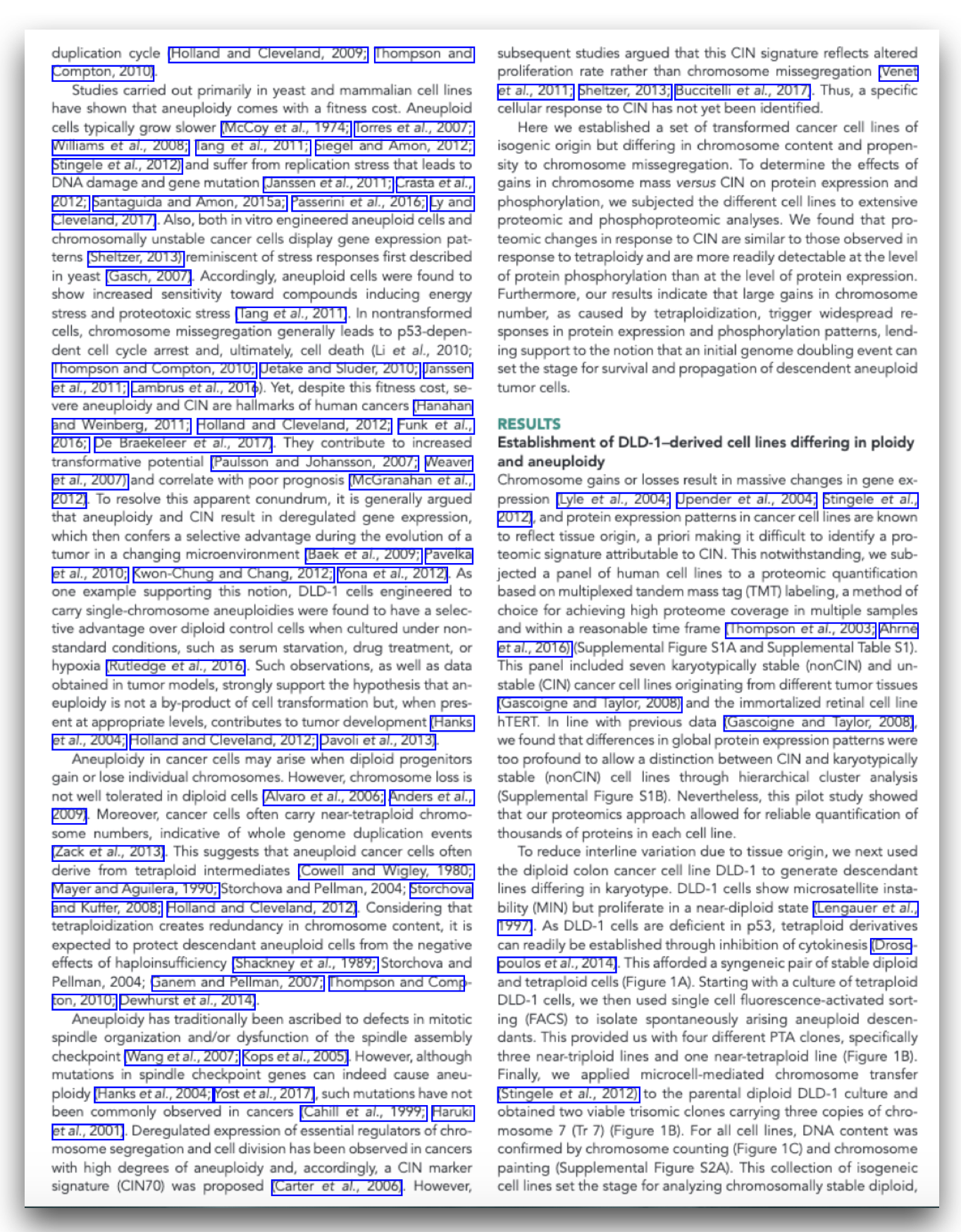
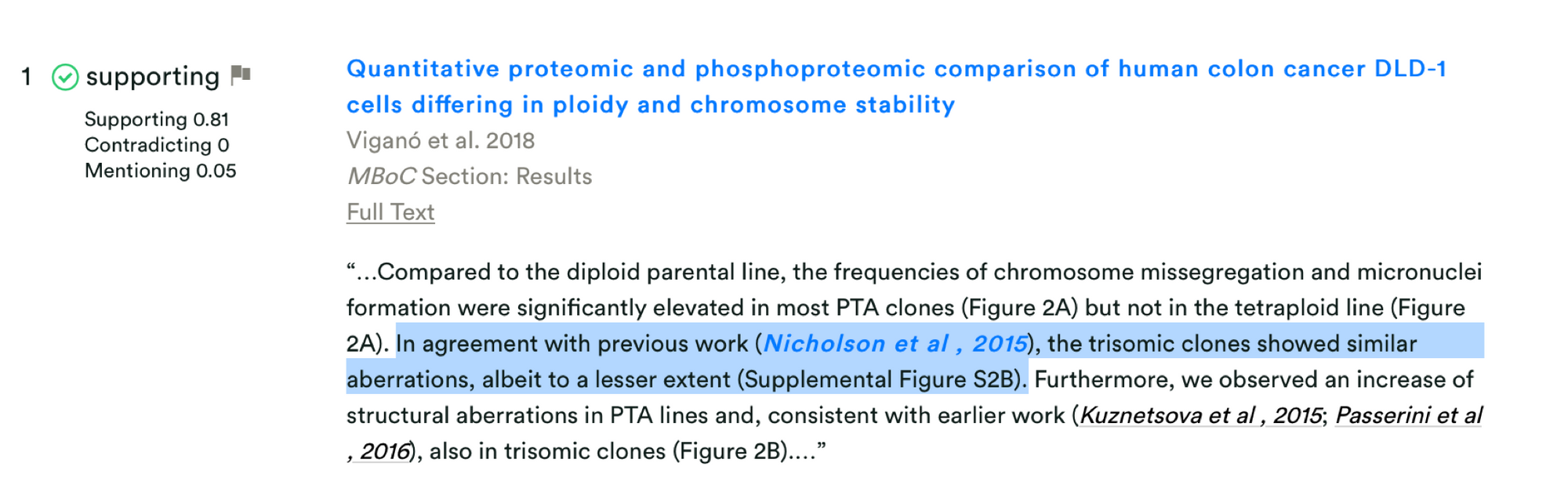
Paperspace: Is your primary model running in production 24/7? How much tuning is going on?
Nicholson: It’s effectively running as much as possible as we are constantly ingesting new articles and analyzing the citation statements we extract from them. We are working on fully automating this process so that as soon as we receive a new article, it is processed and added to the database. Most of this is done in the cloud by CPUs, as well as a GPU that we own and run ourselves.
Paperspace: There’s so much new information coming out everyday about Covid-19. How does scite keep up with the overwhelming amount of inbound data?
Nicholson: At first we didn’t really look at COVID-19 research because I didn’t think we had much to offer. A new paper won’t have citations simply by the fact that it is so new. However, given that people are now publishing new content daily, certain publications are receiving citations on the order of days, whereas it has typically taken months or years. For example, this preprint looking at the severity of COVID-19 in relation to signaling molecules was supported only five days later by another preprint, which we were able to capture with scite.
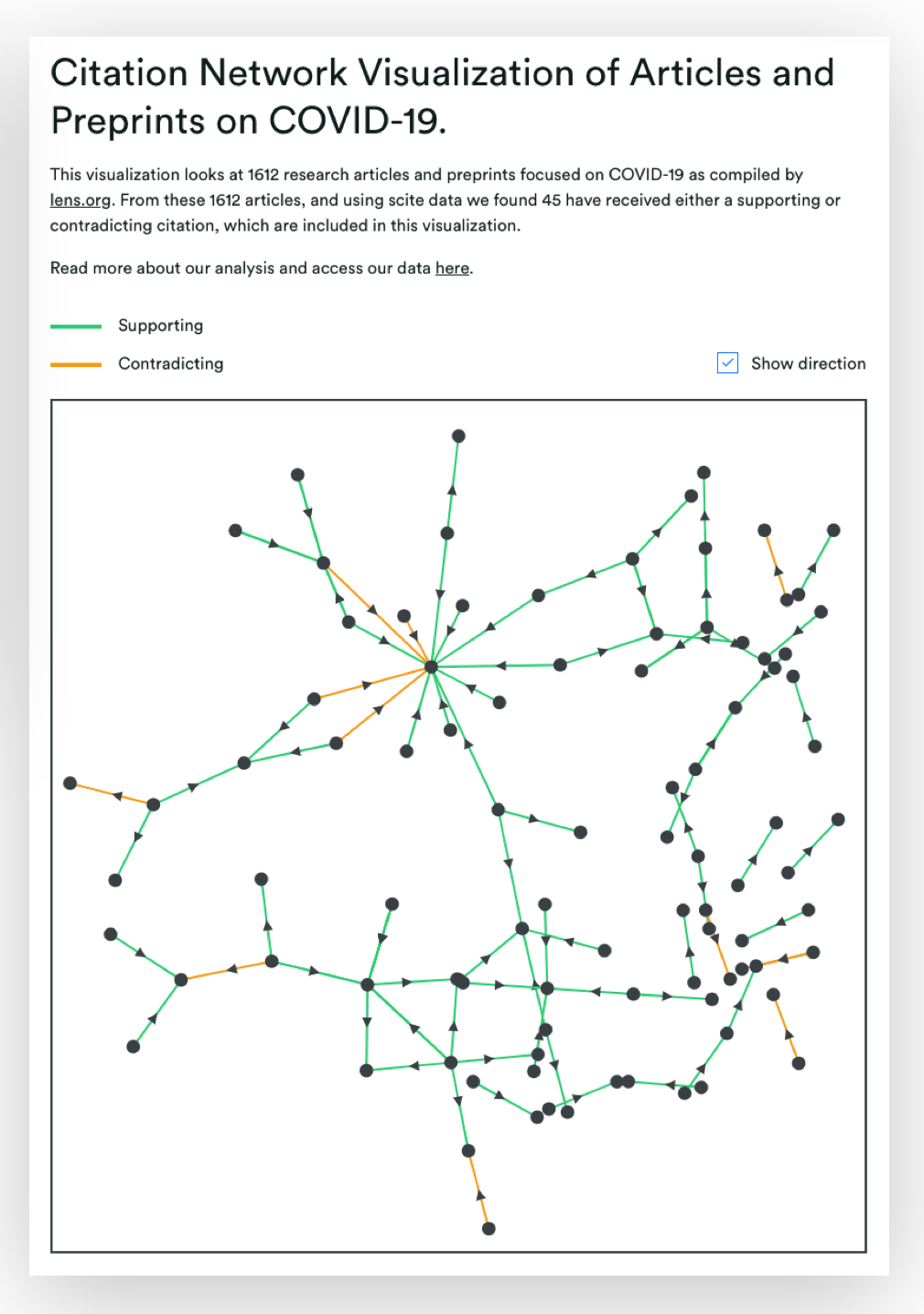
Paperspace: You recently ran every citation in Wikipedia through a machine learning model. What made you want to run this study, and what were your findings?
Nicholson: We decided to do this because Wikipedia is often the first and only stop for many people trying to understand something better. We found that most cited articles (58%) are uncited or untested by subsequent studies, while the remainder show wide variability in contradicting or supporting evidence (2- 40%). This sounds bad, but is actually not too different from all scientific articles in general. Indeed, scientific articles on Wikipedia receive more supporting citations than the scientific literature as a whole.
What we found really interesting was that articles cite references that aren't actually supported in the literature. For example, the Wikipedia article Suicide and Internet states: “A survey has found that suicide-risk individuals who went online for suicide-related purposes, compared with online users who did not report greater suicide-risk symptoms, were less likely to seek help and perceived less social support.” That quote references a scientific report that has been contradicted by another, which is only visible by looking at scite. Having this extra information for this Wikipedia article could influence behavioral choices that have potentially life or death consequences for a large population of people.
Paperspace: How long did it take that model to run?
Nicholson: It takes us about a day to classify about 15M citation statements, but this study was pretty easy for us to do because we took the classifications we had already performed then only looked at articles in Wikipedia.
Paperspace: What is your goal for scite?
Nicholson: I hope that scite will help change how scientists behave, rewarding those who produce strong enough work so that others can validate it as well as rewarding more open debate. Science informs essentially all aspects of our lives and our mission is to make science more reliable.
For more information about scite, please visit: https://scite.ai/