Synthetic media is an exciting new area of research that has seen great advancements in the past few years. This field has the potential to completely revolutionize the way we create and consume content.
This post is a primer on the different types of synthetic media. Then we'll take a deeper look into synthetic video specifically, including its applications and a literature review of recent research that has shaped the field.
What Is Synthetic Media?
Synthetic Media includes artificially-generated video, voice, images or text, where AI takes on part (or all) of the creative process. This falls under the broader landscape of synthetic, artificial or virtual reality (photo-realistic AR/VR). It's a pretty new and exciting space.
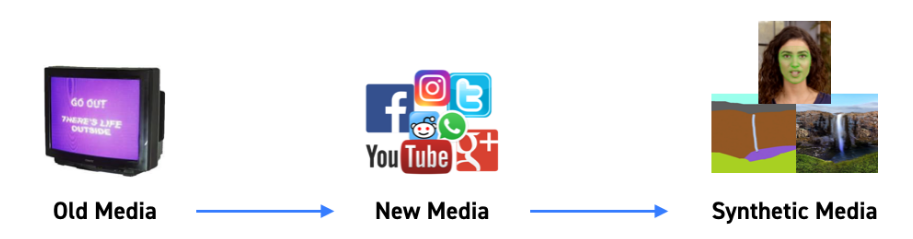
In the past few years there have been significant academic advancements in deep learning, and generative adversarial networks (GANs) have accelerated the growth of synthetic media. This has resulted in the quality of synthetic media improving rapidly, and soon it might just be indistinguishable from traditional media.
Bring this project to life
Why Should You Care?
Because The Future Is Synthetic.
This decade will see a massive proliferation of synthetic media in our daily lives. Synthetic media will bring fundamental shifts in three areas in particular: media creation, licensing and ownership, and verification.
In media creation, synthetic media has the power to completely revolutionize the consumer media landscape and change the media we consume and create. You might wonder, how so? Synthetic media will significantly accelerate creativity, shrinking the gap between idea and content. It will bring with it new methods of communication and storytelling. And it will democratize content production, allowing us to maximize human creativity.
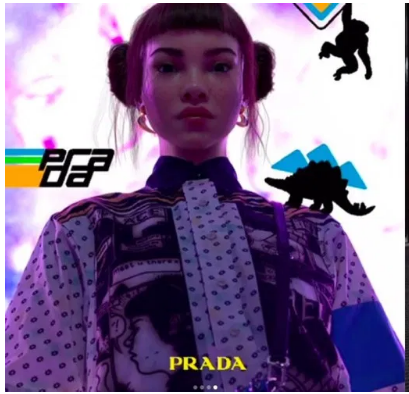
Licensing and ownership will also see a massive change. New laws will have to be made for synthetic videos and voices. Traditionally actors have been paid for their time, physical presence and personal brand. In the not-so-distant future, you'll be able to create a movie starring Brad Pitt without actually having him on set. Who gets paid for this – Brad Pitt, or the techies behind the scenes? And how much?
Companies like Icons8 and Rosebud AI are giving unprecedented power to users, allowing them to create their own diverse, custom photographs in minutes. This removes the need for companies like Shutterstock or Getty Images. Icons8 also has a collection of royalty-free music, generated with AI.
Where there is potential for good, there is also potential for misuse. We need to be careful about this technology and verify all types of media. Companies like Deeptrace and Truepic are dedicated to detecting harmful synthetic videos. This will become more challenging as the lines between what's real and synthetic blur. I imagine a reality where every type of media will be watermarked and fingerprinted, i.e have a verified tag, just like verified accounts on social media.
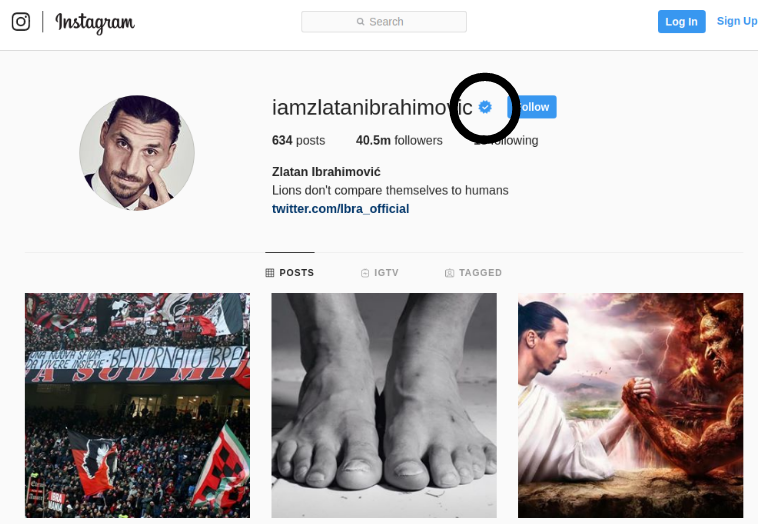
This is an exciting future we’re moving into.
In the next section I'll explore a few popular and noteworthy examples so you get a clear idea of the current synthetic media landscape in 2020.
Examples and Applications
Synthetic Reality
Lil Miquela and other Virtual Avatars. Lil Miquela is the world’s most popular virtual influencer withs 1.8 million followers on Instagram. She shoots ads for brands like Calvin Klein and Loreal, has appeared in videos with Bella Hadid and J Balvin (who are real celebrities), and she also has her own music videos. However, she is not real. Lil Miquela is a 3D model created by a team of virtual effects artists from Brud. Virtual influencers like her (it?) have become extremely famous and will continue to rise in popularity.
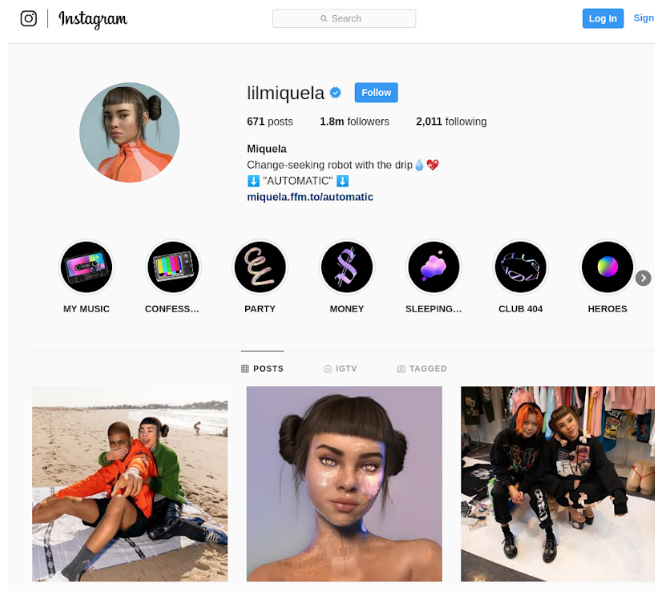
Animation/Gaming and Mixed Reality
AI tools to create and edit 2D and 3D animations are on the rise and in great demand, given how fast the gaming and mixed reality markets are growing. They have the potential to revolutionize the process behind creating characters, scenes and other animated/virtual elements. RADiCAL is one such company that allows users to create a 3D animation of themselves just from iPhone videos. You would typically need a very expensive motion-capture rig, with a bodysuit and motion capture cameras, to produce what RADiCAL offers. Imagine being able to upload your own dances to Fortnite.
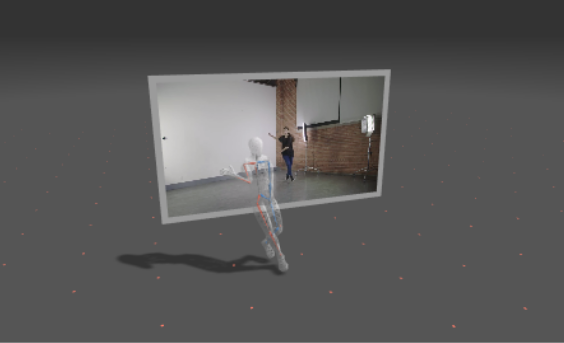
Synthetic Videos
The most popular type of synthetic videos are currently Deepfakes. These are essentially face swaps, where one person’s face replaces another's (like Nicolas Cage’s face on Donald Trump). This is done using GANs. It’s unfortunate that Deepfakes have become almost infamous, since they can also be used for a lot of good. Here’s a Deepfake of Salvador Dali greeting people at the Dali Museum in St Petersburg, Florida. Something like this would normally require you to hire a very expensive CGI studio, but the only expense here are from developers and GPUs.
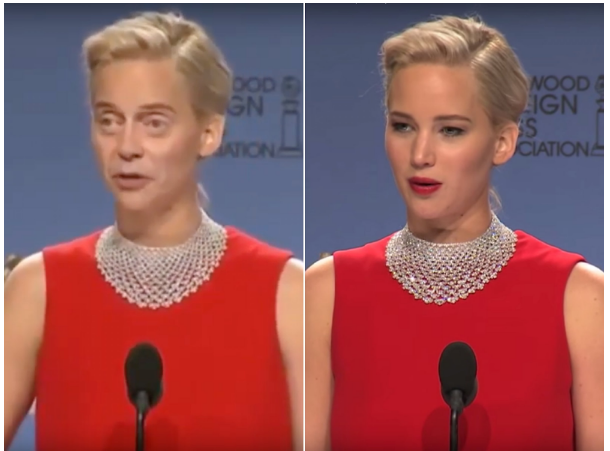
Another type of synthetic video involves facial reenactment, where a source actor controls a target actor’s face. This gives us different world leaders singing Imagine by John Lenon and David Beckham speaking 9 different languages.
Synthetic Images
Images (along with text) are one the earliest types of synthetic media, with Pix2Pix and CycleGAN making splashes in the deep learning community back in 2016 and 2017. Some of the popular examples of their application include Edmond De Belamy, a painting created by Obvious AI which sold for almost half a million dollars, and artificiallt generated stock images which are making companies like Shutterstock and Getty Images obsolete.
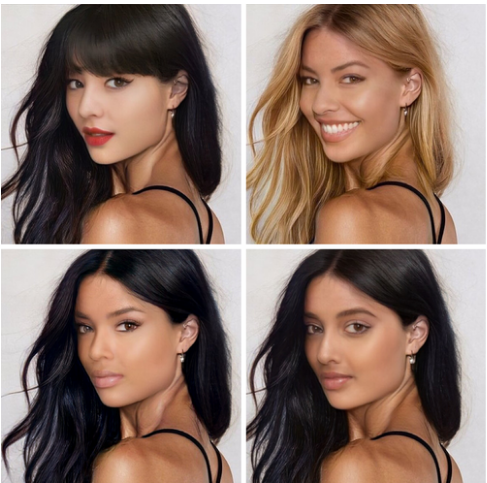
Synthetic Audio
The world is seeing a proliferation in different forms of audio tech, from podcasts to smart speakers. However, it takes time, money and effort (voice artists, studios, microphones and processing, etc.) to record anything. This has made artificial voice technology like text-to-speech (TTS) and voice cloning very popular. For example, Resemble.ai is a popular company that allows you to clone your own voice for creating digital avatars.
Another closely related field is synthetic music. Companies like Popgun.ai and Jukedeck (recently acquired by TikTok) help users create music using AI. Tech like this will help everyone sing, play instruments, compose songs and master audio, thereby truly democratizing music creation.
I hope you now have a decent understanding of the synthetic media landscape and the applications of each type of artificially generated media. I will be focusing on synthetic video, images and audio in this series of articles, and here I will focus on synthetic video in particular. In the next section I will review important papers which shaped this field.
Synthetic Video - Applications and Research Review
I am going to cover some of the applications and examples in Synthetic Video and summarize a few of the papers that make this tech possible. This is not an exhaustive list, but a list of applications and papers that I feel are the most significant ones.
Applications and Publications Covered
1. Face Swap (DeepFakes)
2. Facial Reenactment from Video
3. Facial Reenactment from Speech/Audio
- Synthesizing Obama: Learning Lip Sync from Audio
- Neural Voice Puppetry: Audio-driven Facial Reenactment
4. Full Body Reenactment
Face Swap (Deepfakes)
Face Swap (DeepFakes) are essentially Face Swap models. Most of the popular open-source ones are Autoencoder based (SAE, HAE etc) and there are very few that are based on GANs.
What Is the Technology Behind This?
There are no formal or seminal papers on DeepFakes since they have not come out of any lab. Here’s an explanation behind the tech though. Let’s take the popular Jennifer Buscemi example. Say we have videos of both of them, Jennifer Lawrence and Steve Buscemi. We want to put Steve Buscemi’s face (Face B) onto Jennifer Lawrence’s Face (Face A). We have 2 autoencoders, one for each of them.
Training
The two autoencoders are trained separately, both of them share the same encoder but have different decoders. The common encoder is trained with both the faces, but the Decoder of Face A is only trained with faces of A; the Decoder B is only trained with faces of B.
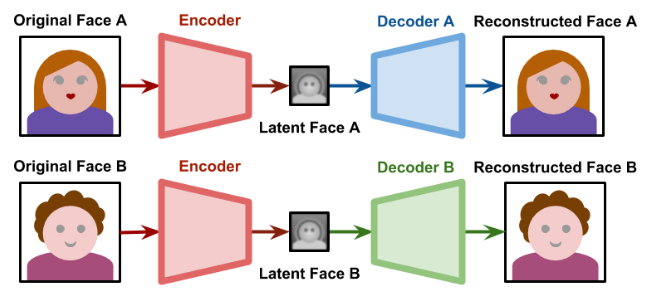
Generation
Now for generating a DeepFake where Steve Buscemi’s face (face B) is on Jennifer Lawrence’s face (face A), we pass a video with Jennifer Lawrence into the encoder and instead of trying to reconstruct her face from the encoding, we now pass it to Decoder B to reconstruct Steve’s Buscemi’s face. The end result is a video of Steve Buscemi’s Face on Jennifer Lawrence.
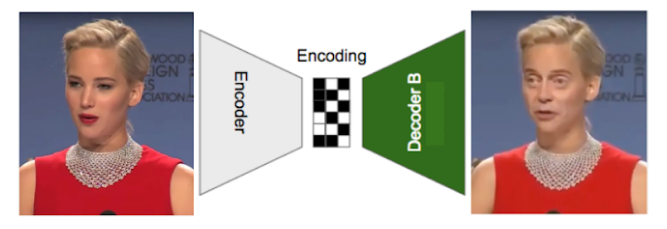
NOTE: DeepFakes have gained a decent amount of notoriety and are illegal in some parts of the world (for instance, DeepFakes were banned in China) – arguably with good reason. I feel it’s important to list a few efforts that have been made to detect DeepFakes:
- https://deepfakedetectionchallenge.ai/
- FaceForensics
- DeepTrace → Biggest name working in this space, founded by a bunch of UvA grads.
Facial Reenactment and Visual Dubbing from Video
Facial Reenactment is the process of controlling a target actor’s face using a source actor’s (It’s actually controlling a Face using any source, where the vector can be a Face, audio or text. I cover reenactment driven by a video in this section and by audio in the next). Face reenactment models transfer the pose, rotation and expressions from one face to another. This enables a variety of applications, ranging from creating photo-realistic virtual avatars, allowing celebrities to talk in multiple languages seamlessly and the power to disrupt the video production industry.
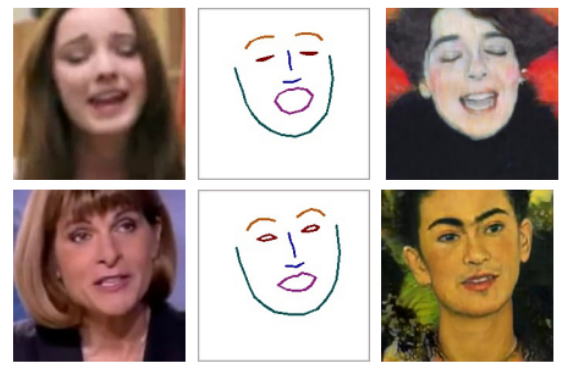
What is Visual Dubbing?
Visual dubbing is a particular instance of face reenactment that aims to alter the mouth motion of the target actor to match a new audio track, commonly spoken in a foreign language by a dubbing actor. This is primarily used in making dubbing better by syncing the lips of an actor to the dubbed voice. The best example of this is Syntheisa.io making Beckham speak 9 languages.
Visual dubbing can be performance-driven (where the target is controlled by the movement’s of a source actor) or speech-driven (Audio driven).
Face2Face: Real-time Face Capture and Reenactment of RGB Videos (CVPR 2016) [Paper]
Facial reenactment has been an interest of the Computer Vision community for quite some time, and was commonly achieved using RDB-D sensors (Like Kinect) or using a marker setup. This paper from TUM’s Visual Computing group in 2016 was the first to perform real-time facial reenactment of monocular target video (e.g., Youtube video) using a source sequence which is also a monocular video stream, captured live with a commodity webcam. The goal was to animate the facial expressions of the target video by a source actor and re-render the manipulated output video in a photo-realistic fashion.
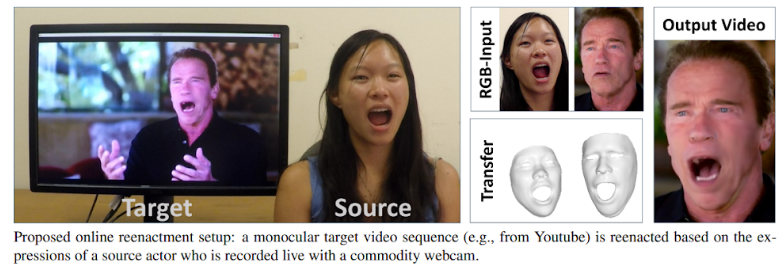
Approach
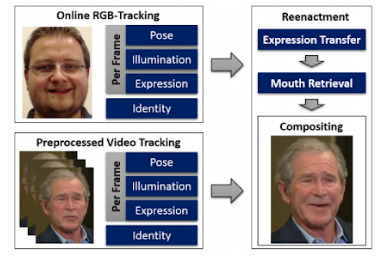
A PCA model is used to parameterize the face. The first two dimensions represent facial identity – i.e., geometric shape and skin reflectance – and the third dimension controls the facial expression.
The model first reconstructs the shape identity of the target actor.
- Both source and target videos are processed by the model, and the reenactment is achieved by fast and efficient deformation transfer between the source and the target. From the target sequence, the best-match mouth interior is retrieved and warped to produce an accurate fit.
- For creating the final image, they re-render the target’s face with the transferred expressions (using the transferred expression coefficients) and composite it (blend it) with the target video’s background. The re-rendering also takes into account the estimated lighting in the target scene. (Refer the paper for Energy/Loss formulas)
The mouth area which is the lips and teeth are usually difficult to synthesize, resulting in inconsistent renders. They overcome this by introducing a new mouth synthesis approach that generates a realistic mouth interior by retrieving and warping best matching mouth shapes from the offline sample sequence and they maintain the appearance of the target mouth shape.
Results
This paper shows highly-realistic reenactment examples on a variety of target Youtube videos at a resolution of 1280×720. The results are qualitative.
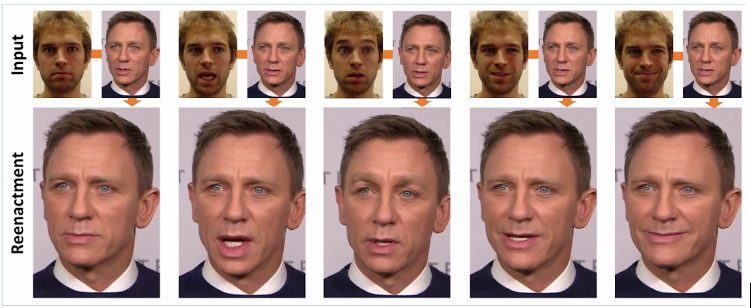
Here’s a video with more results.
Deep Video Portraits (SIGGRAPH 2019) [arxiv]
This is an interesting paper that made waves in the community back in 2018. They are the first to transfer the full 3D head pose, face expression, eye gaze and eye blinking, as compared to other approaches that were restricted to manipulations of facial expressions. They were also the first to synthesize photo-realistic videos of the target person’s upper body, including realistic clothing and hair. (A video portrait is a video with a person’s head and upper body). The core of this approach is a generative neural network with a novel space-time architecture. (Anything space-time deals with video data since a video is essentially images over time). The network takes as input synthetic renderings of a parametric face model, based on which it predicts photo-realistic video frames for a given target actor.
In Face2Face, only the face expression can be modified realistically, but not the full 3D head pose, nor do they have a consistent upper body/consistently changing background.
Approach
The paper formulates video portrait synthesis and reenactment as a rendering-to-video translation task.
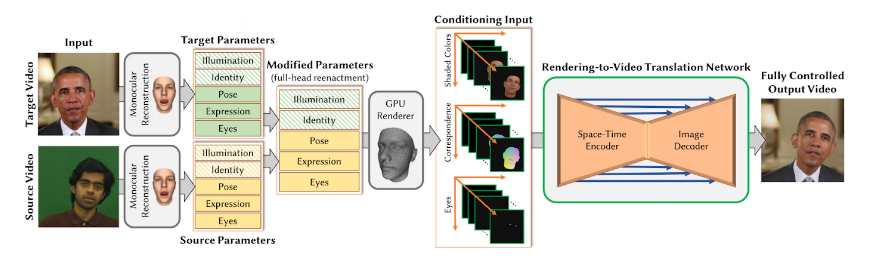
First, the source and target actors are tracked using a face reconstruction approach that uses a parametric face and illumination model. The resulting sequence of low dimensional parameter vectors represents the actor’s identity, head pose, expression, eye gaze, and the scene lighting for every video frame. This allows them to transfer the head pose, expression, and/or eye gaze parameters from the source to the target.
In the next step they generate new synthetic renderings of the target actor based on the modified parameters. Three different conditioning inputs are generated: a color rendering, a correspondence image, and an eye gaze image.
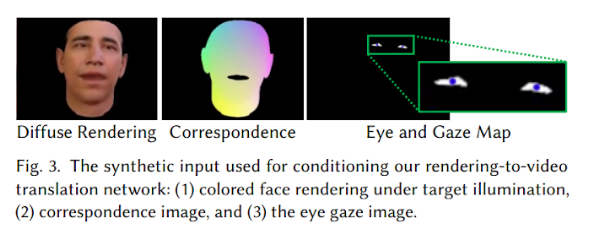
These renderings serve as conditioning input to their novel rendering-to-video translation network , which is trained to convert the synthetic input into photo-realistic outputs.
At the core of the approach is a conditional generative adversarial network (cGAN) which is specifically tailored to video portrait synthesis. The Generator of the cGAN is a rendering-to-video translation network. The rendering-to-video translation network is a space time encoder (i.e video encoder) with an image decoder that takes the new synthetic renderings as conditions. The rendering-to-video transfer is trained in an adversarial manner.
The discriminator D tries to get better at classifying given images as real or synthetic, while the rendering-to-translation network T tries to improve in fooling the discriminator
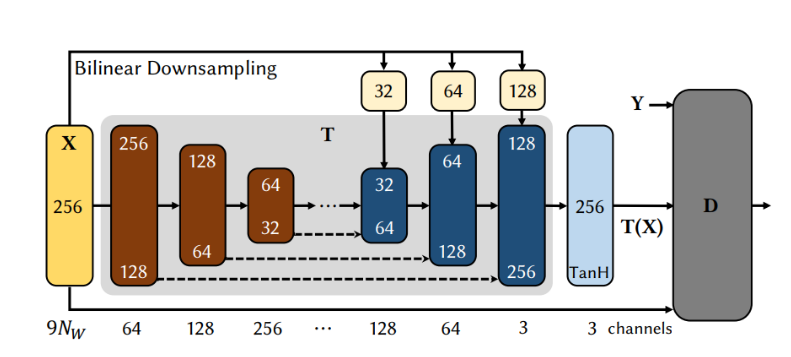
Results
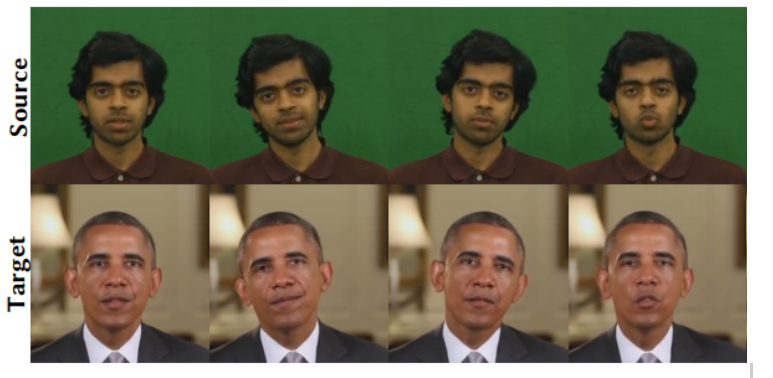
Have a look at the project website for more qualitative results.
Here are a few other papers that I find interesting.
- VDub: Modifying Face Video of Actors for Plausible Visual Dubbing (EUROGRAPHICS 2015) [arxiv]
- ReenactGAN: Learning to Reenact Faces via Boundary Transfer (ECCV 2018) [arxiv] [CODE]
- Deferred Neural Rendering: Image Synthesis using Neural Textures (2019) [arxiv]
- Few-Shot Adversarial Learning of Realistic Neural Talking Head Models (ICCV 2019) [arxiv] [CODE]
- Realistic Speech-Driven Facial Animation with GANs (2019) [arxiv]
Facial Reenactment from Speech/Audio
Facial Animation from text or speech is basically controlling a face using text or speech. Strictly speaking this falls under Facial Reenactment, but for the sake of simplicity I’ve divided it into categories based on what the source driver is - The source can be a video of a source actor (or) in this case audio.
This problem of generating a mouth video from audio is pretty difficult, due to (1) the technical challenge of mapping a one-dimensional signal to a (3D) time-varying image, (2) but also due to the fact that humans are extremely attuned to subtle details in the mouth region.
Facial Reenactment from Speech/Audio was first introduced by Bregler et al. who demonstrated how to “rewrite” a person’s lip movement in a video to match a new audio track represented as a phoneme sequence. Since then there has been quite a bit of progress and I cover 2 important papers.
Synthesizing Obama: Learning Lip Sync from Audio (2017) [arxiv] [code]
This is a fairly straightforward paper compared to the papers in the previous section. This was quite an influential one too. Supasorn (the first author) ended up giving a TED talk on his work.
Given audio of President Barack Obama, this paper synthesizes a high-quality video of him speaking with accurate lip sync, composited into a target video clip. They trained a recurrent neural network on many hours (17 hrs) of his weekly address footage and the network learns the mapping from raw audio features to mouth shapes. Using the mouth shape at each time instant, they synthesize high-quality mouth texture, and composite (blend) it with proper 3D pose.
Approach
The approach is based on synthesizing video from audio in the region around the mouth and using compositing techniques to borrow the rest of the head and torso from other stock footage. A recurrent neural network is used for synthesizing mouth shape from audio which was trained on millions of video frames.
The input audio track is the source, and the target video is a stock video clip into which they composite the synthesized mouth region
To make the problem easier, the paper focuses on synthesizing parts of the face that are most correlated to speech. For the Presidential address footage, the content of Obama’s speech correlates most strongly to the region around the mouth (lips, cheeks, and chin), and also aspects of head motion – his head stops moving when he pauses his speech. They, therefore, focus on synthesizing the region around his mouth, and borrow the rest of Obama (eyes, head, upper torso, background) from stock footage.
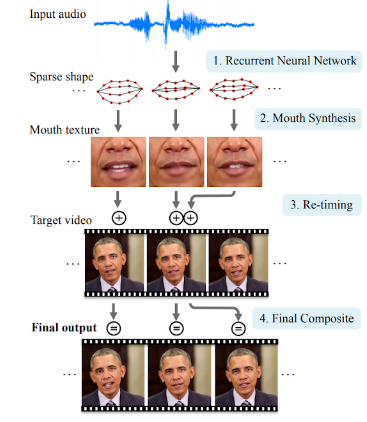
The Overall pipeline works as follows (As shown in the figure):
- Given an audio of Obama, audio features are extracted to use as input to a recurrent neural network that outputs, for every output video frame, a sparse mouth shape
- From the sparse mouth shape, texture for the mouth and lower region of the face are synthesized. The mouth texture is then blended onto a stock video that is modified so that the head motion appears natural and matches with the given input speech
- During blending, the jawline is warped to match the chin of the new speech, and the face is composed to a target frame in the original pose.
Results
Look at this video for qualitative results.
Neural Voice Puppetry: Audio-driven Facial Reenactment (2019) [arxiv]
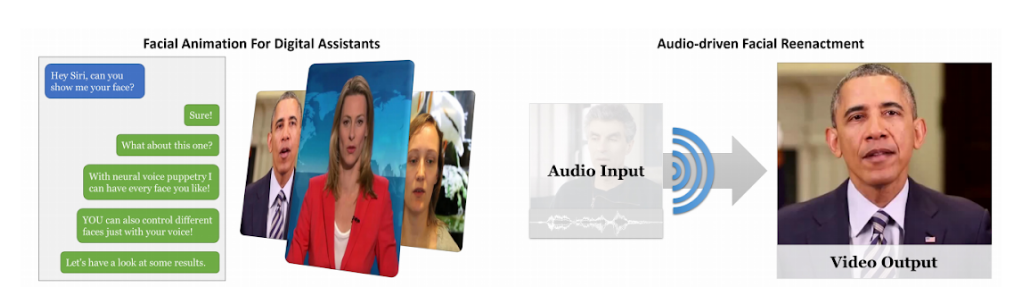
This paper came out in December 2019 and is the current SOTA for audio-driven Facial Reenactment. Their approach generalizes across different people, allowing them to synthesize videos of a target actor with the voice of any unknown source actor or even synthetic voices that can be generated utilizing standard text-to-speech approaches. Neural Voice Puppetry is an easy to use audio-to-video translation tool which does not require vast amount of video footage of a single target video or any manual user input. The target videos are comparably short (2-3 min) which is awesome.
Method
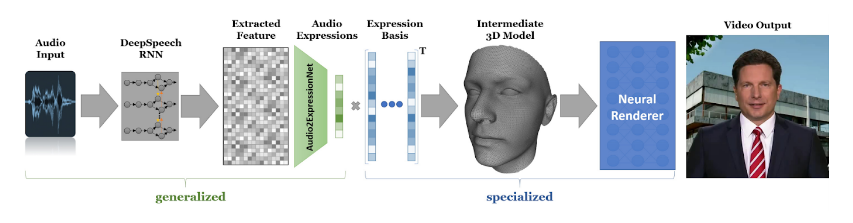
The Neural Voice Puppetry pipeline consists of two main parts - A generalized Network and a specialized network.
The generalized network (Audio2Expression Net) predicts a latent expression vector and spans an audio-expression space. To ensure generalization among multiple persons, the latent audio expression space is shared by all persons. The audio expressions are interpreted as blendshape coefficients of a 3D face model rig. This face model rig is person-specific and is optimized in the second part of the pipeline. The dataset for training the Audio2ExpressionNet consists of 116 videos with an average length of 1.7min (in total 302750 frames). The training corpus is selected such that the persons are in a neutral mood (commentators of the German public TV).
The specialized stage is the second stage and captures the idiosyncrasies of a target person i.e facial motion and appearance. Since every person has its own talking style and, thus, different expressions, the paper establishes person-specific expression spaces that can be computed for every target sequence. The space is trained on a short video sequence of 2−3 minutes (in comparison to hours that are required by state-of-the-art methods).
Facial reenactment is achieved by mapping from the audio expression-space (first stage) to the person-specific expression space (second stage). Given the estimated expression (reenactment) and the extracted audio features, a novel deferred neural rendering technique is applied that generates the final output image. (Look at this paper for more info on neural textures and Deferred Neural Renderings)
Since the audio-based expression estimation network is generalized among multiple persons, it can be applied to unseen actors. However, the person-specific rendering network for the new target video is trained from scratch.
Results
The results are heavily qualitative so it’s best to have a look at the videos on their webpage. Here are a few figures.
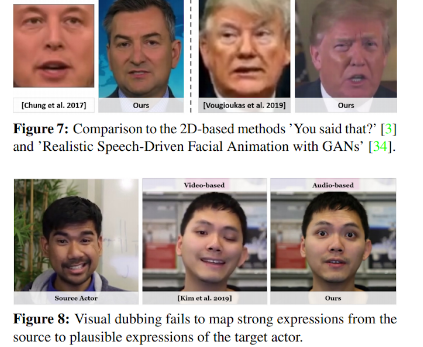
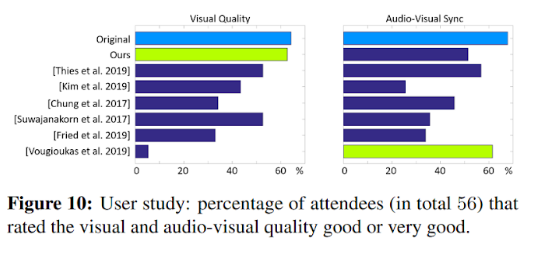
This paper demonstrates shows superior visual and lip sync quality compared to others and is the current SOTA.
Here are more interesting papers and a few with code implementations.
- You said that? - Synthesizing videos of talking faces from audio (2017) - Seminal paper in audio driven Facial Reenactment [arxiv] [code]
- ATVGnet - Hierarchical Cross-modal Talking Face Generation with Dynamic Pixel-wise Loss (CVPR 2019) [arxiv][CODE]
- Text-based Editing of Talking-head Video (2019) [arxiv] - Brilliant paper from Stanford that allows you to edit the audio in a video and make a new video altogether. You can expect to see this tech in After Effects very soon.
Full-Body Reenactment
There doesn’t seem to be a formal name to this yet, so I’m calling it Full-body Reenactment. It is similar to Facial Reenactment, however here the complete body of a target actor is reenacted in a photo-realistic manner driven by a source.
LumièreNet: Lecture Video Synthesis from Audio (2019) [arxiv]
This is a very interesting paper from Udacity AI that generates photo-realistic videos of an Instructor from audio. Instructor-produced lecture videos are very popular in MOOCs, but shooting a video requires considerable resources and processes (i.e., instructor, studio, equipment, and production staff) and takes a lot of time. What if we can generate a new Lecture video from existing footage, driven by text or audio? This will make video production very agile and there is no need to re-shoot each new video
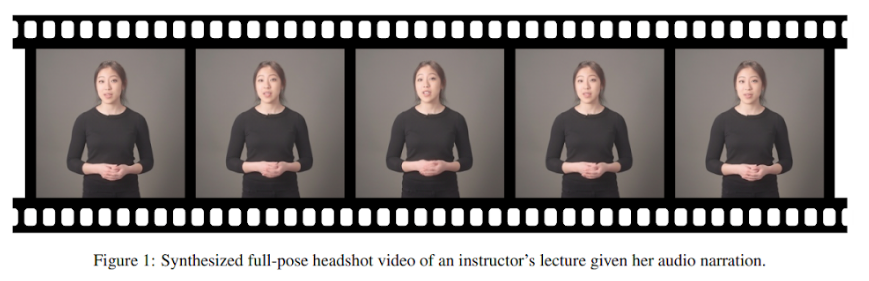
Approach
They introduce a pose estimation based latent representation as an intermediate code to synthesize an instructor’s face, body, and the background altogether. They design these compact and abstract codes from the extracted human poses for a subject.
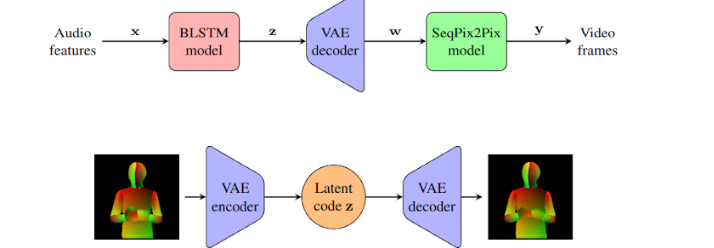
LumièreNet consists of three neural network modules: the BLSTM model, the VAE model, and the SeqPix2Pix model.
- The BLSTM model first associates extracted audio features x to intermediate latent codes z.
- Then, the VAE decoder constructs the corresponding pose figures w from z.
- Lastly, the SeqPix2Pix model produces the final video frames y given w.
- During training, LumièreNet learns the VAE model to design compact and abstract latent codes z for high-dimensional DensePose images altogether with both encoder and decoder.
Results
Have a look this video for qualitative results. The results aren’t the best, with noticeable audio-visual discrepancies. However it’s a very good addition to the EdTech space and I’m excited to see what comes next.
Everybody Dance Now (ICCV 2018) [arxiv] [code]
This popular paper presents a simple method for “do as I do” motion transfer: given a source video of a person dancing, they transfer that performance to a target after only a few minutes of the target subject performing standard moves. They approach this problem as video-to-video translation using pose as an intermediate representation. To transfer the motion, poses are extracted from the source subject and learned pose-to-appearance mappings are applied to generate the target subject.
Two consecutive frames are predicted for temporally coherent video results and a separate pipeline for realistic face synthesis is introduced (FaceGAN). Although the method is quite simple, it produces surprisingly compelling results (see video).
Method
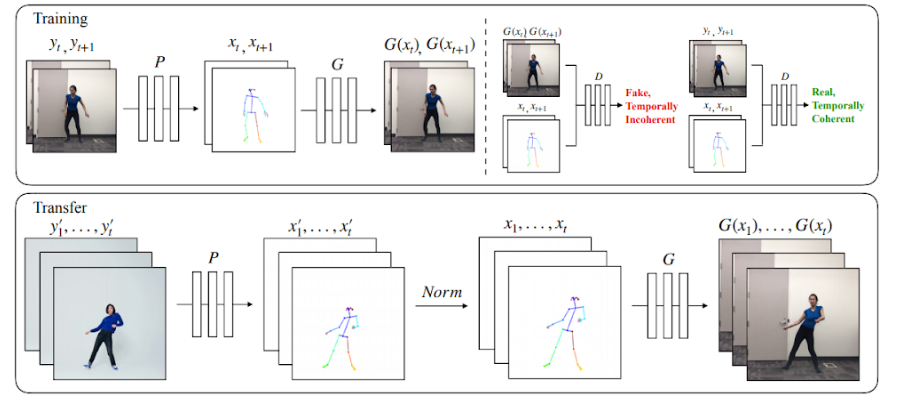
Training - The model uses a pose detector P to create pose stick figures from video frames of the target subject. The mapping G is learnt alongside an adversarial discriminator D which attempts to distinguish between the “real” correspondences (xt, xt+1),(yt, yt+1) and the “fake” sequence (xt, xt+1),(G(xt), G(xt+1)) .Transfer - A pose detector P is used to obtain pose joints for the source person that are transformed by the normalization process (Norm) into joints for the target person for which pose stick figures are created. Then the trained mapping G is applied.
Transfer - A pose detector P is used to obtain pose joints for the source person that are transformed by the normalization process (Norm) into joints for the target person for which pose stick figures are created. Then the trained mapping G is applied.
Qualitative Results
More interesting papers:
- Video-to-Video Synthesis (2018) [arxiv] [code]
- Speech2Gesture: Learning Individual Styles of Conversational Gesture (2019) [arxiv] [code]
I’m super excited about the future we’re moving into. Comment/Reach out to me if you have any questions!
Further Reading
- https://www.axios.com/synthetic-realities-fiction-stories-fact-misinformation-ed86ce3b-f1a5-4e7b-ba86-f87a918d962e.html
- Stanford Course on Computational Video Manipulation - Excellent Resource
- https://betaworksventures.com/campv1 - First Synthetic Media accelerator of sorts. Very exciting stuff.