Bring this project to life
In the Gen-AI world, you can now experiment with different hairstyles and create a creative look for yourself. Whether contemplating a drastic change or simply seeking a fresh look, the process of imagining oneself with a new hairstyle can be both exciting and daunting. However, with the use of artificial intelligence (AI) technology, the landscape of hairstyling transformations is undergoing a groundbreaking revolution.
Imagine being able to explore an endless array of hairstyles, from classic cuts to 90's designs, all from the comfort of your own home. This futuristic fantasy is now a possible reality thanks to AI-powered virtual hairstyling platforms. By utilizing the power of advanced algorithms and machine learning, these innovative platforms allow users to digitally try on various hairstyles in real-time, providing a seamless and immersive experience unlike anything seen before.
In this article, we will explore HairFastGAN and understand how AI is revolutionizing how we experiment with our hair. Whether you're a beauty enthusiast eager to explore new trends or someone contemplating a bold hair makeover, join us on a journey through the exciting world of AI-powered virtual hairstyles.
Introduction
This paper introduces HairFast, a novel model designed to simplify the complex task of transferring hairstyles from reference images to personal photos for virtual try-on. Unlike existing methods that are either too slow or sacrifice quality, HairFast excels in speed and reconstruction accuracy. By operating in StyleGAN's FS latent space and incorporating enhanced encoders and inpainting techniques, HairFast successfully achieves high-resolution results in near real-time, even when faced with challenging pose differences between source and target images. This approach outperforms existing methods, delivering impressive realism and quality, even when transferring hairstyle shape and color in less than a second.
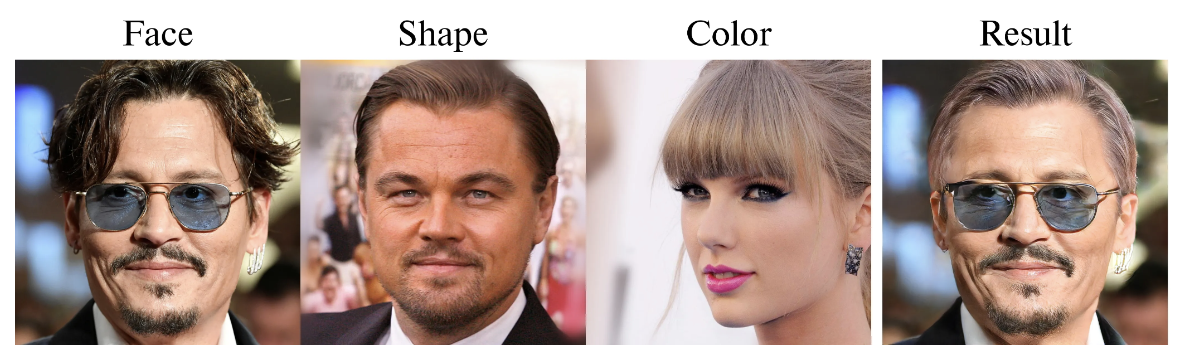
Thanks to advancements in Generative Adversarial Networks (GANs), we can now use them for semantic face editing, which includes changing hairstyles. Hairstyle transfer is a particularly tricky and fascinating aspect of this field. Essentially, it involves taking characteristics like hair color, shape, and texture from one photo and applying them to another while keeping the person's identity and background intact. Understanding how these attributes work together is crucial for getting good results. This kind of editing has many practical uses, whether you're a professional working with photo editing software or just someone playing virtual reality or computer games.
The HairFast method is a fast and high-quality solution for changing hairstyles in photos. It can handle high-resolution images and produces results comparable to the best existing methods. It's also quick enough for interactive use, thanks to its efficient use of encoders. This method works in four steps: embedding, alignment, blending, and post-processing. Each step is handled by a special encoder trained to do that specific job.
Related Works
Recent advancements in Generative Adversarial Networks (GANs), like ProgressiveGAN, StyleGAN, and StyleGAN2, have greatly improved image generation, particularly in creating highly realistic human faces. However, achieving high-quality, fully controlled hair editing remains a challenge due to various complexities.
Different methods address this challenge in different ways. Some focus on balancing editability and reconstruction fidelity through latent space embedding techniques, while others, like Barbershop, decompose the hair transfer task into embedding, alignment, and blending subtasks.
Approaches like StyleYourHair and StyleGANSalon aims for greater realism by incorporating local style matching and pose alignment losses. Meanwhile, HairNet and HairCLIPv2 handle complex poses and diverse input formats.
Encoder-based methods, such as MichiGAN and HairFIT, speed up runtime by training neural networks instead of using optimization processes. CtrlHair, a standout model, utilizes encoders to transfer color and texture, but still faces challenges with complex facial poses, leading to slow performance due to inefficient postprocessing.
Overall, while significant progress has been made in hair editing using GANs, there are still hurdles to overcome for achieving seamless and efficient results in various scenarios.
Methodology Overview
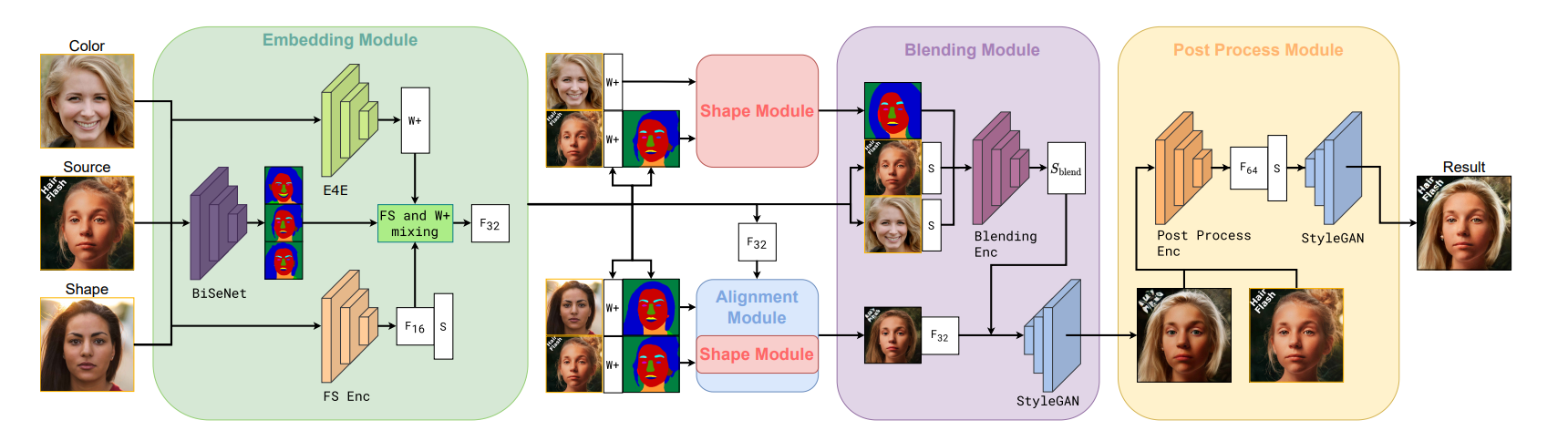
This novel method for transferring hairstyles is very similar to the Barbershop approach however—all optimization processes are replaced with trained encoders for better efficiency. In the Embedding module, original images representation are captured in StyleGAN spaces, like W+ for editing and F S space for detailed reconstruction. Additionally, face segmentation masks are used for later use.
Moving to the Alignment module, the shape of the hairstyle from one image to another is mainly done by focusing on changing the tensor F. Here, two tasks are completed: generating the desired hairstyle shape via the Shape Module and adjusting the F tensor for inpainting post-shape change.
In the Blending module, the shift of hair color from one image to another is done . By editing the S space of the source image using the trained encoder, this is achieved while considering additional embeddings from the source images.
Although the image post-blending could be considered final, a new Post-Processing module is required. This step aims to restore any lost details from the original image, ensuring facial identity preservation and method realism enhancement.
Embedding
To start changing a hairstyle, first images are converted into StyleGAN space. Methods like Barbershop and StyleYourHair do this by reconstructing each image in F S space through an optimization process. Instead, in this research a pre-trained FS encoder is used that quickly gives the F S representations of images. It's one of the best encoders out there and makes images look really good.
But here's the issue: F S space isn't easy to work with. When changing hair color using the FS encoder in Barbershop, it doesn't do a great job. So, another encoder called E4E is used. It's simple and not as good at making images look nice, but it's great for making changes. Next, the F tensor (which holds the information about the hair) from both encoders is mixed to solve this problem.
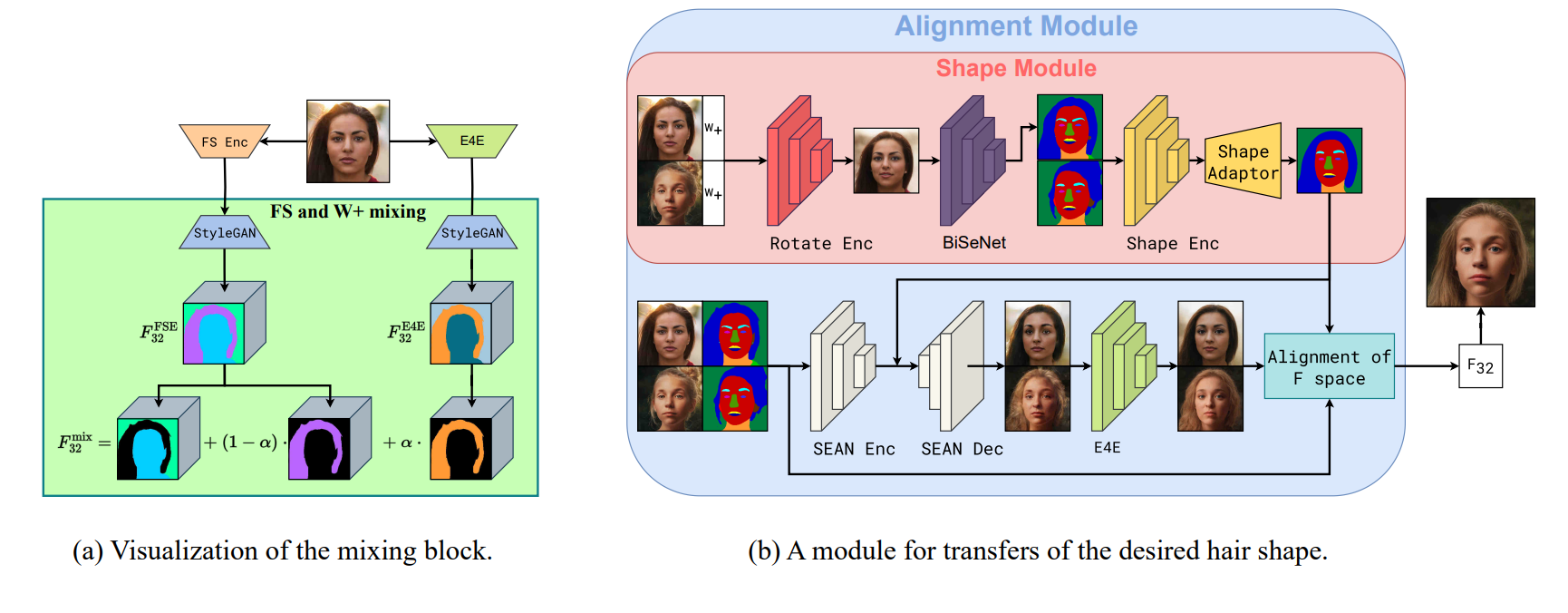
Alignment
In this step, the hair makeover is done, so the hair in one picture should look like the hair in another picture. To do this, a mask is created that outlines the hair, and then the hair in the first picture is adjusted to match that mask.
Some smart folks came up with a way to do this called CtrlHair. They use a Shape Encoder to understand the shapes of hair and faces in pictures and a Shape Adaptor to adjust the hair in one picture to match the shape of another. This method usually works pretty well, but it has some issues.
One big problem is that the Shape Adaptor is trained to handle hair and faces in similar poses. So if the poses are really different between the two pictures, it can mess things up, making the hair look weird. The CtrlHair team tried to fix this by tweaking the mask afterwards, but it's not the most efficient solution.
To tackle this issue, an additional tool called Rotate Encoder was developed. It's trained to adjust the shape image to match the pose of the source image. This is mainly done by tweaking the representation of the image before segmenting it. There is no need to fine-tune the details for creating the mask, so a simplified representation is used in this case. This encoder is trained to handle complex poses without distorting the hair. If the poses already match, it won't mess up the hairstyles.
Blending
In the next step, the main focus is on changing the hair color to the desired shade. Previously, as we know Barbershop's previous method that was too rigid, trying to find a balance between the source and desired color vectors. This often resulted in incomplete edits and added unwanted artifacts due to outdated optimization techniques.
To improve this, a similar encoder architecture called HairCLIP is added predicts how the style of the hair vector changes when given two input vectors. This method uses special modulation layers that are more stable and great for changing styles.
Additionally, we're feeding our model with CLIP embeddings of both the source image (including hair) and the hair-only part of the color image. This extra information helps preserve details that might get lost during the embedding process and has been shown to significantly enhance the final result, according to our experiments.
Experiments Results
The experiments revealed that while the CtrlHair method scored the best according to the FID metric, it actually didn't perform as well visually compared to other state-of-the-art approaches. This discrepancy occurs due to its post-processing technique, which involved blending the original image with the final result using Poisson blending. While this approach was favored by the FID metric, it often resulted in noticeable blending artifacts. On the other hand, the HairFast method had a better blending step but struggled with cases where there were significant changes in facial hues. This made it challenging to use Poisson blending effectively, as it tended to emphasize differences in shades, leading to lower scores on quality metrics.
A novel post-processing module has been developed in this research, which is like a supercharged tool for fixing images. It's designed to handle more complex tasks, like rebuilding the original face and background, fixing up hair after blending, and filling in any missing parts. This module creates a really detailed image, with four times more detail than what we used before. Unlike other tools that focus on editing images, ours prioritizes making the image look as good as possible without needing further edits.
Demo
Bring this project to life
To run this demo we will first, open the notebook HairFastGAN.ipynb
. This notebook has all the code we need try and experiment with the model. To run the demo, we first need to clone the repo and install the necessary libraries however.
- Clone the repo and install Ninja
!wget https://github.com/ninja-build/ninja/releases/download/v1.8.2/ninja-linux.zip
!sudo unzip ninja-linux.zip -d /usr/local/bin/
!sudo update-alternatives --install /usr/bin/ninja ninja /usr/local/bin/ninja 1 --force
## clone repo
!git clone https://github.com/AIRI-Institute/HairFastGAN
%cd HairFastGAN
- Install some necessary packages and the pre-trained models
from concurrent.futures import ProcessPoolExecutor
def install_packages():
!pip install pillow==10.0.0 face_alignment dill==0.2.7.1 addict fpie \
git+https://github.com/openai/CLIP.git -q
def download_models():
!git clone https://huggingface.co/AIRI-Institute/HairFastGAN
!cd HairFastGAN && git lfs pull && cd ..
!mv HairFastGAN/pretrained_models pretrained_models
!mv HairFastGAN/input input
!rm -rf HairFastGAN
with ProcessPoolExecutor() as executor:
executor.submit(install_packages)
executor.submit(download_models)
- Next, we will set up an argument parser, which will create an instance of the
HairFast
class, and perform hair swapping operation, using default configuration or parameters.
import argparse
from pathlib import Path
from hair_swap import HairFast, get_parser
model_args = get_parser()
hair_fast = HairFast(model_args.parse_args([]))
- Use the below script which contains the functions for downloading, converting, and displaying images, with support for caching and various input formats.
import requests
from io import BytesIO
from PIL import Image
from functools import cache
import matplotlib.pyplot as plt
import matplotlib.gridspec as gridspec
import torchvision.transforms as T
import torch
%matplotlib inline
def to_tuple(func):
def wrapper(arg):
if isinstance(arg, list):
arg = tuple(arg)
return func(arg)
return wrapper
@to_tuple
@cache
def download_and_convert_to_pil(urls):
pil_images = []
for url in urls:
response = requests.get(url, allow_redirects=True, headers={"User-Agent": "Mozilla/5.0"})
img = Image.open(BytesIO(response.content))
pil_images.append(img)
print(f"Downloaded an image of size {img.size}")
return pil_images
def display_images(images=None, **kwargs):
is_titles = images is None
images = images or kwargs
grid = gridspec.GridSpec(1, len(images))
fig = plt.figure(figsize=(20, 10))
for i, item in enumerate(images.items() if is_titles else images):
title, img = item if is_titles else (None, item)
img = T.functional.to_pil_image(img) if isinstance(img, torch.Tensor) else img
img = Image.open(img) if isinstance(img, str | Path) else img
ax = fig.add_subplot(1, len(images), i+1)
ax.imshow(img)
if title:
ax.set_title(title, fontsize=20)
ax.axis('off')
plt.show()
- Try the hair swap with the downloaded image
input_dir = Path('/HairFastGAN/input')
face_path = input_dir / '6.png'
shape_path = input_dir / '7.png'
color_path = input_dir / '8.png'
final_image = hair_fast.swap(face_path, shape_path, color_path)
T.functional.to_pil_image(final_image).resize((512, 512)) # 1024 -> 512
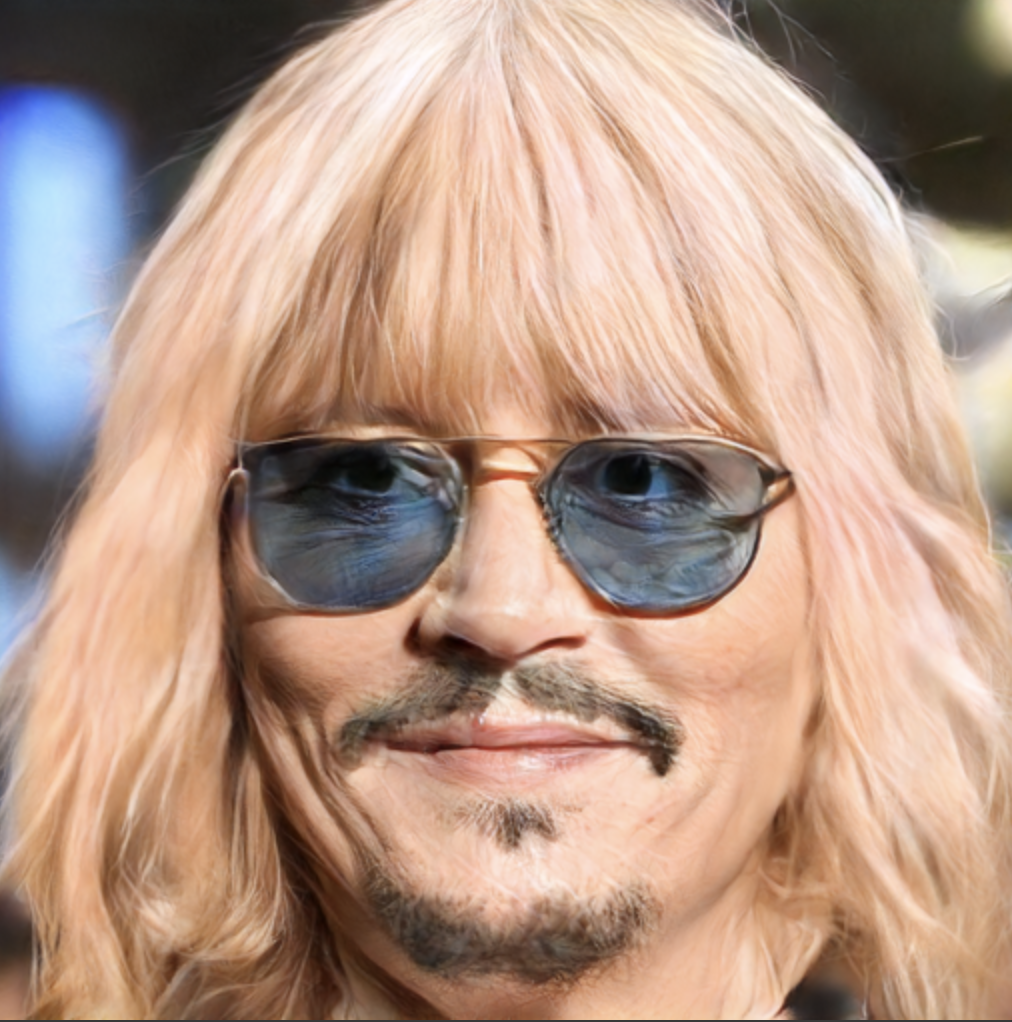
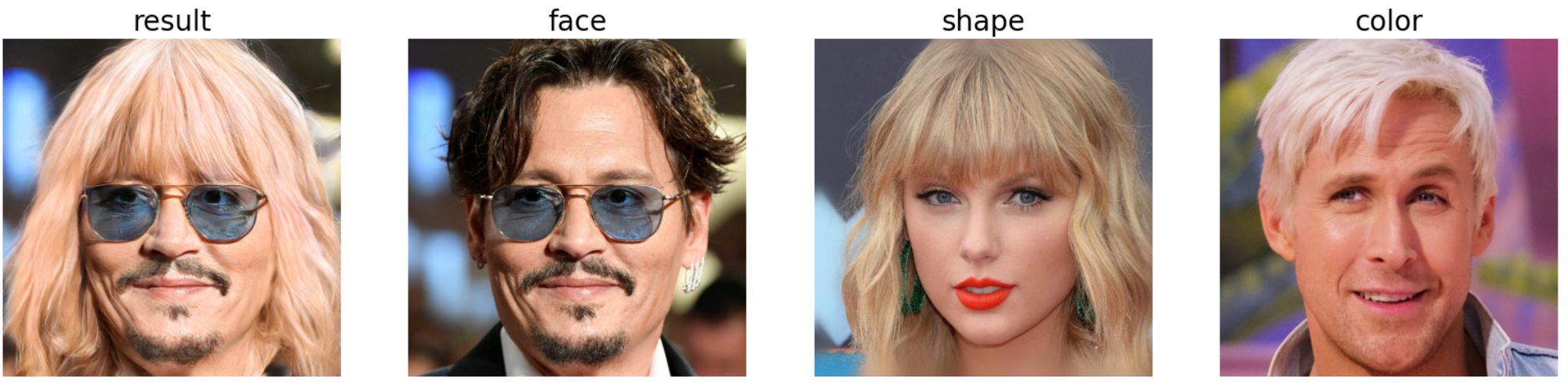
Ending Thoughts
In our article, we introduced the HairFast method for transferring hair, which stands out for its ability to deliver high-quality, high-resolution results comparable to optimization-based methods while operating at nearly real-time speeds.
However, like many other methods, this method is also constrained by the limited ways to transfer hairstyles. Yet, the architecture lays the groundwork for addressing this limitation in future work.
Furthermore, the future of virtual hair styling using AI holds immense promise for revolutionizing the way we interact with and explore hairstyles. With advancements in AI technologies, even more realistic and customizable virtual hair makeover tools are expected. Hence, this leads to highly personalized virtual styling experiences.
Moreover, as the research in this field continues to improve, we can expect to see greater integration of virtual hair styling tools across various platforms, from mobile apps to virtual reality environments. This widespread accessibility will empower users to experiment with different looks and trends from the comfort of their own devices.
Overall, the future of virtual hair styling using AI holds the potential to redefine beauty standards, empower individuals to express themselves creatively and transform the way we perceive and engage with hairstyling.
We enjoyed experimenting with HairFastGAN's novel approach, and we really hope you enjoyed reading the article and trying it with Paperspace.
Thank You!